|
 |

Current research topics:
|
|
Video Smoke Detection Using Deep Neural Networks Enhanced with Synthetic Smoke Plume Generation (PDF) |
|
|
Qixing Zhang, Gao Xu, Gaohua Lin, Kewei Wang, and Yongming Zhang
|
|
|
Video smoke detection is a promising fire detection method especially in open or lager spaces and outdoor environments.
However, there is still room for improvement of the recognition rate. In recent years, great success in the field of image
recognition has been achieved by deep learning, which relies on large scale training data. Unfortunately, due to the
occasionality of fire accidents, it is difficult and expensive to obtain a large number of fire smoke video samples
used to train the deep neural networks.
Our completed work and in progress work will be presented in this poster accepted by 12th IAFSS symposium. The
Symposium will be held on June 12-16,2017 at Lund University, Sweden.
|
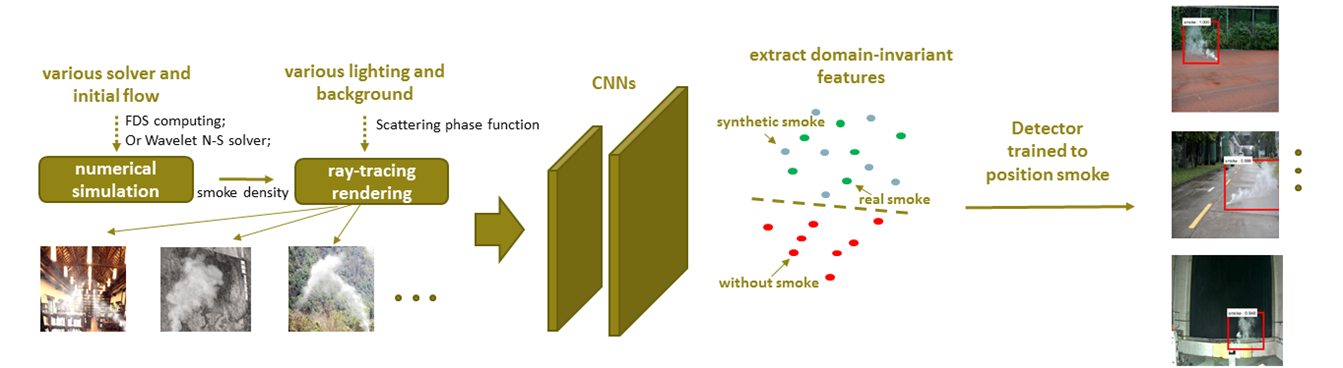 |
|
|
|
|
|
|
|
|
Deep Domain Adaptation Based Video Smoke Detection using Synthetic Smoke Images, preprint in arxiv (PDF) |
|
|
Gao Xu,Yongming Zhang,Qixing Zhang,Gaohua Lin,Jinjun Wang
|
|
|
In this paper, a deep domain adaptation based method for video smoke detection is proposed
to extract a powerful feature representation of smoke. Due to the smoke image samples limited
in scale and diversity for deep CNN training, we systematically produced adequate synthetic
smoke images with a wide variation in the smoke shape, background and lighting conditions.
Considering that the appearance gap (dataset bias) between synthetic and real smoke images
degrades significantly the performance of the trained model on the test set composed fully
of real images, we build deep architectures based on domain adaptation to confuse the
distributions of features extracted from synthetic and real smoke images. This approach
expands the domain-invariant feature space for smoke image samples. With their approximate
feature distribution off non-smoke images, the recognition rate of the trained model is improved
significantly compared to the model trained directly on mixed dataset of synthetic and real
images. Experimentally, several deep architectures with different design choices are applied to
the smoke detector. The ultimate framework can get a satisfactory result on the test set. We
believe that our approach is a start in the direction of utilizing deep neural networks enhanced
with synthetic smoke images for video smoke detection.
|
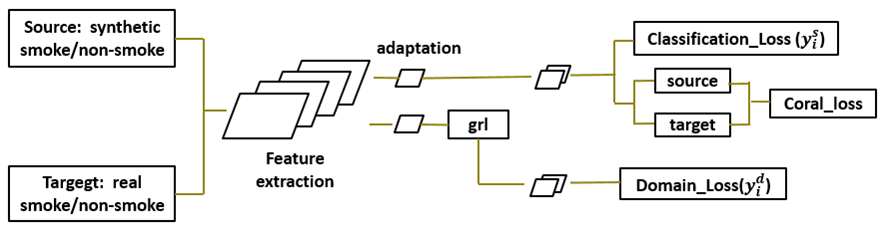 |
|
|
|
|
|
|
Smoke detection in video sequences based on dynamic texture using volume local binary patterns (PDF) |
|
|
Gaohua Lin, Yongming Zhang, Qixing Zhang, Yang Jia, Gao Xu and Jinjun Wang
|
|
|
In this paper, a video based smoke detection method using dynamic texture feature extraction with
volume local binary patterns is studied. Block based method was used to distinguish smoke frames
in high definition videos obtained by experiments firstly. Then we propose a method that directly
extracts dynamic texture features based on irregular motion regions to reduce adverse impacts of
block size and motion area ratio threshold. Several general volume local binary patterns were used
to extract dynamic texture, including LBPTOP, VLBP, CLBPTOP and CVLBP, to study the effect of the
number of sample points, frame interval and modes of the operator on smoke detection. Support vector
machine was used as the classifier for dynamic texture features. The results show that dynamic
texture is a reliable clue for video based smoke detection. It is generally conducive to reducing
the false alarm rate by increasing the dimension of the feature vector. However, it does not always
contribute to the improvement of the detection rate. Additionally, it is found that the feature
computing time is not directly related to the vector dimension in our experiments, which is important
for the realization of real-time detection. |
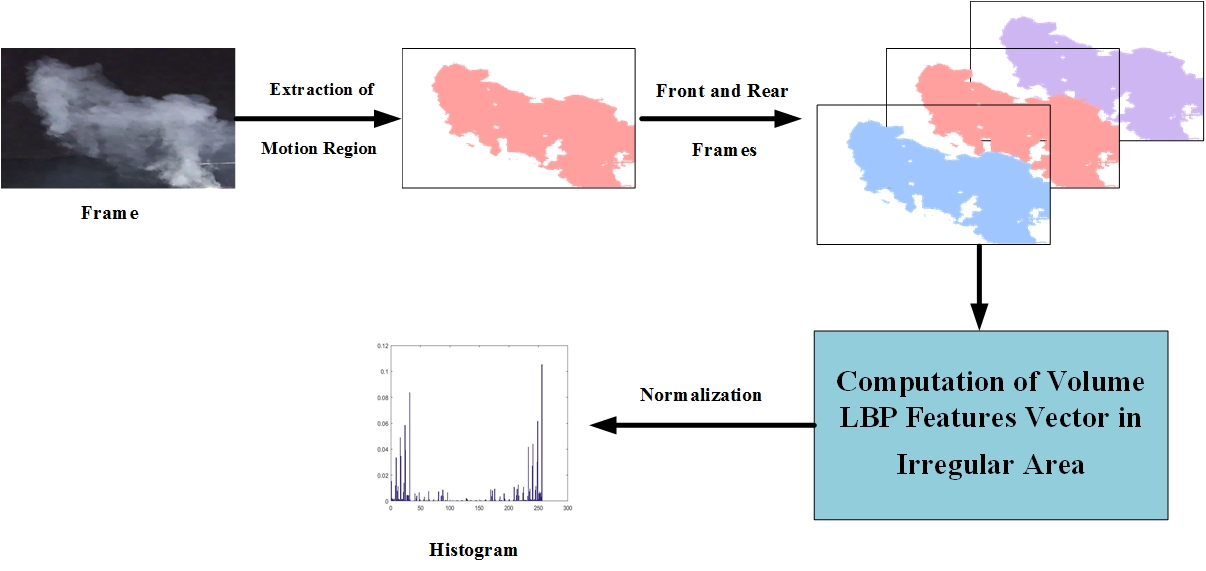 |
|
|
|
|
|
|
Video Fire Detection using thermal infrared and visible images |
|
|
Qixing Zhang, Gaohua Lin, Gao Xu, et al.
|
|
|
The research is financially supported by the Anhui Provincial Key R&D Program (1704a0902030) and the Fundamental Research Funds for the Central Universities (WK6030000029).
The research is aimed to design a video fire detection system based on simultaneously processing of thermal infrared and visible images.
|
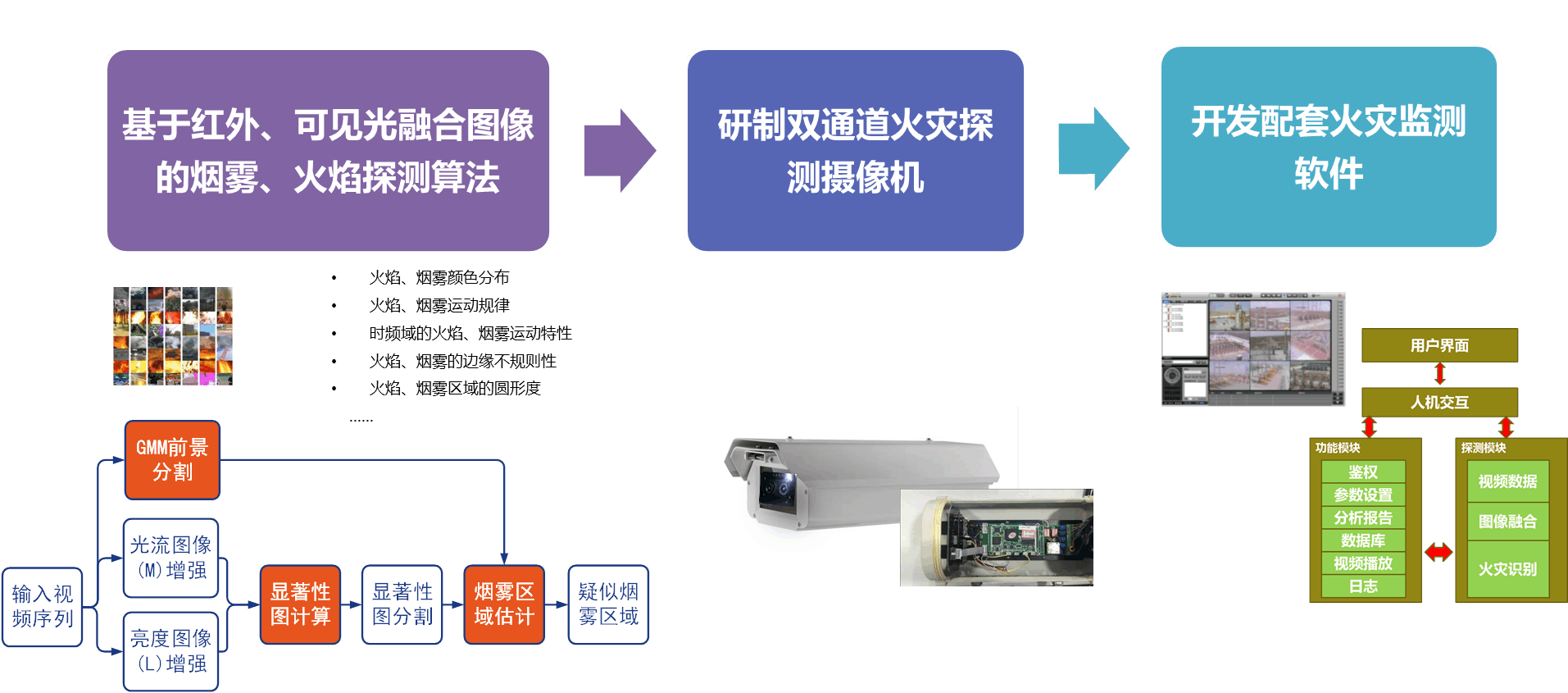 |
|
|
|
|
|
|
|
|